Four steps for using MAXQDA’s AI Assist in the coding process of a qualitative research project
The analysis of qualitative data such as interviews, focus groups, reports or field notes has undergone significant methodological and technological development over the last thirty years. The use of qualitative data analysis software to implement research methods such as qualitative content analysis (Kuckartz & Rädiker 2023), grounded theory (e.g. Strauss & Corbin 1990), thematic analysis (Brown & Clark 2021) has become common practice in a wide range of fields.
We are now seeing the proliferation of a new technology that has the potential to transform the methods used in qualitative research: the use of generative AI. As a new technology, it is both a driver of innovation and a major source of concern, particularly for teachers and other educators. As recently discussed as part of a symposium titled “Opportunities and Challenges of AI in Research” at the MAXQDA user conference MQIC 2024, there is a lot of uncertainty about how the use of generative AI might affect qualitative research and its methods. Will AI-based analysis replace coding? Or will the transparency and explicitness of coding become even more important with the newly introduced ambiguity of AI tools?
In this blog post we will take a look at how to combine the potential of generative AI and coding, not only to reflect on the possibilities, but also to provide a step-by-step introduction on how to use generative AI in a qualitative research project that relies heavily on the technique of ‘coding’.
What is AI Assist and how does AI Coding work?
MAXQDA is an established qualitative data analysis software that has been used for qualitative and mixed methods research for over three decades. With the release of the “AI Assist” add-on in April 2023, the software incorporated the latest technological advances in the field of generative AI. With the release of AI Assist, we see the fusion of the powerful capabilities of generative AI models with a proven research platform, mainly centred around the technique of ‘coding’ data.
As an add-on to MAXQDA, AI Assist offers a wide range of options, from creating summaries of documents, to chatting with coded data, to suggesting code ideas for the research process. All of these features follow the principle of an “assistant” that helps in many small steps along the process, rather than promising to take over the entire research project. Among these features is a new feature called “AI Coding”, which by its very name promises to bridge the gap between generative AI and coding.
However, in the spirit of an “assistant”, AI Coding does not code the entire research project autonomously. Instead, the software has a very specific purpose: to apply a single, well-described code to our data. Given a code name and a code description (in the form of a code memo), the AI assistant attempts to identify text segments within a document according to the code description.
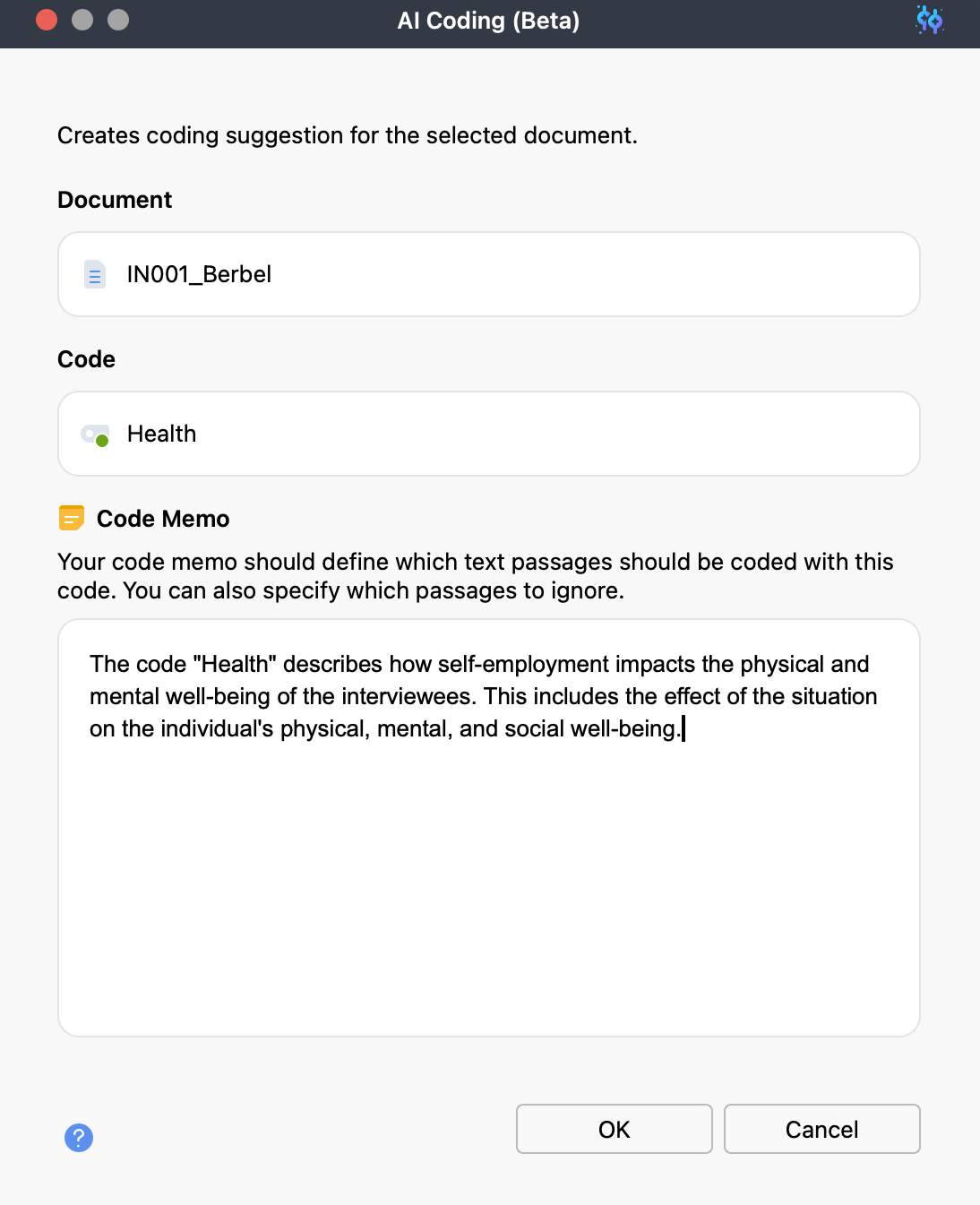
Example: Interface AI Coding
This code-by-code, document-by-document approach may require a few extra clicks, but it allows for a tightly controlled process. Once executed, the AI identifies units of meaning by creating its own data segments, codes them with the appropriate code, and provides a brief explanation describing how the identified text segment relates to the code.
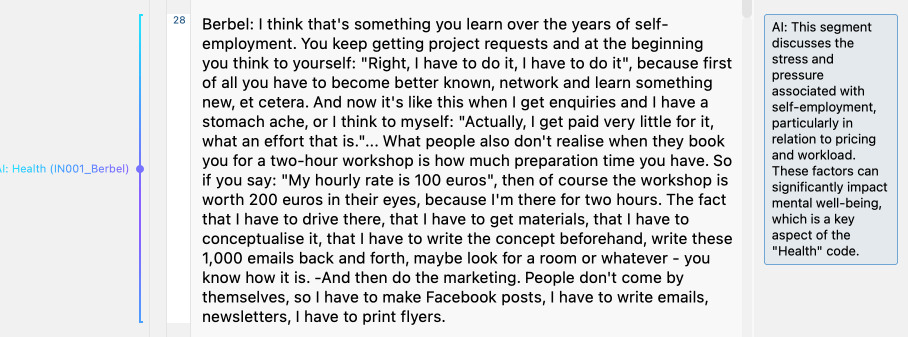
Example: AI explanation of the segments as segment comment
This helps to make the process more transparent and may also provide inspiration for developing subcodes later on.
In addition, the newly created AI code is stored as a subcode of the original code. In this way, AI codes are clearly identified and kept separate from our other codes. The researcher can then review the results and decide whether to refine the code, discard it or integrate it into the existing code.
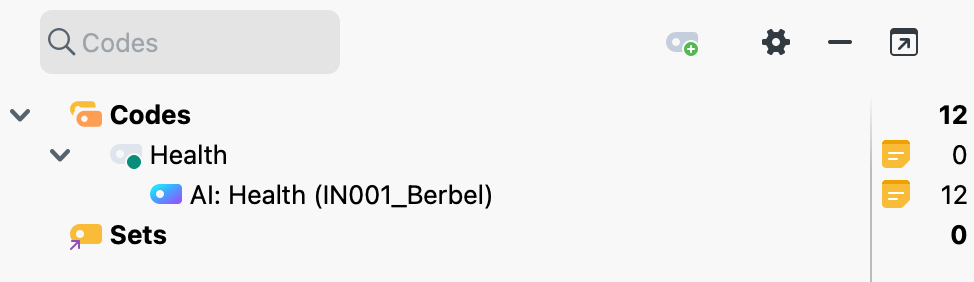
AI Code has been created as subcode of the original code.
So how do we use a feature like AI Assist as part of a research process? Let’s look at this as a four-step process:
- Developing and testing the code descriptions on a subset of the data
- Applying the codes to the full dataset
- Validating the AI coding using visual tools
- Analysing the AI coded data
Step 1: Developing and testing code descriptions using a subset of the data
If we look at this new functionality, we can see that it has been created in the image of a technique popular in qualitative research: Concept-driven or ‘deductive’ coding (Kuckartz & Rädiker 2023). And it raises an important question: How do we develop a good, unambiguous code description to guide our coding? No matter how good the AI is, it will always be limited by the quality of our code description. So let’s first look at how to set up a code and code notes for AI coding, and then consider what makes a good code note for AI coding.
How do I create the description for AI Coding in MAXQDA?
To use AI Coding, we first need to create a code. To do this, simply click on the green plus sign in MAXQDA’s code window. A window will open:
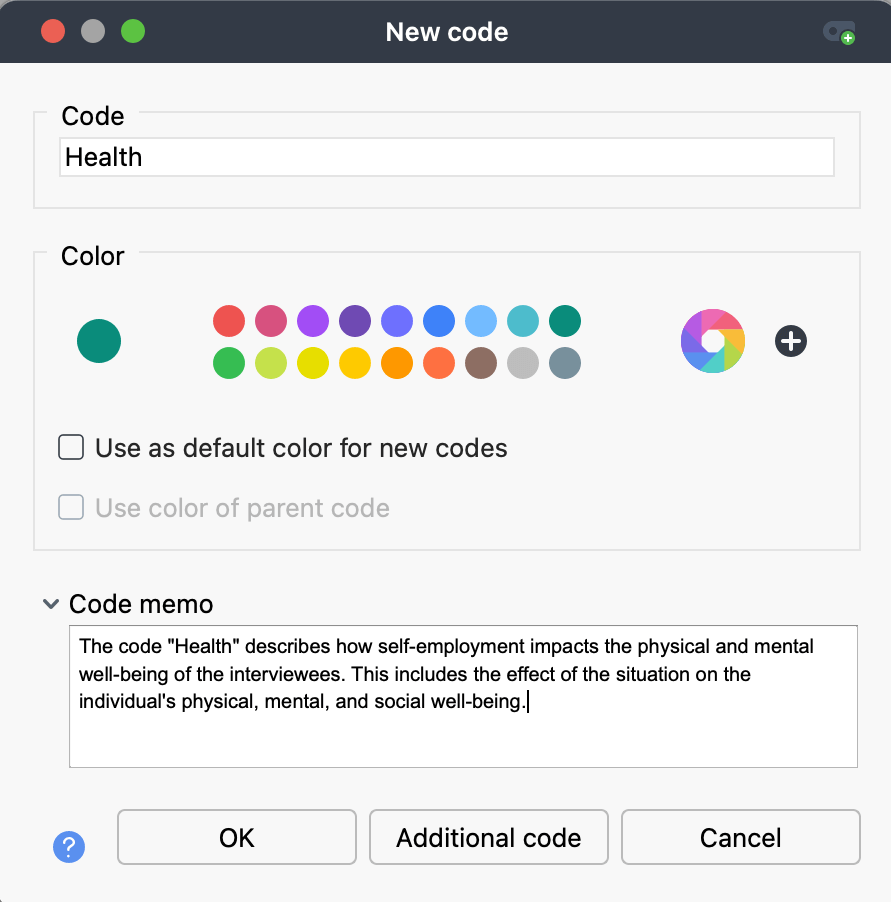
Creating a new Code “Health”.
In the first field of the window, we are asked to enter a name for the code. We can also choose a color. In the second text field we can enter a description of the code in the code memo. This is important because both the code name and the code memo will be taken into account when applying our code with the help of the AI.
Once we have created our code, all we need to do is open the document we want to code. Then select the AI Coding option from the “AI Assist > AI Coding” menu:

The “AI Assist” Tab
Here we select the appropriate code by dragging and dropping it into the “Code” field. Then we have another opportunity to revise our code memo before the code is applied:
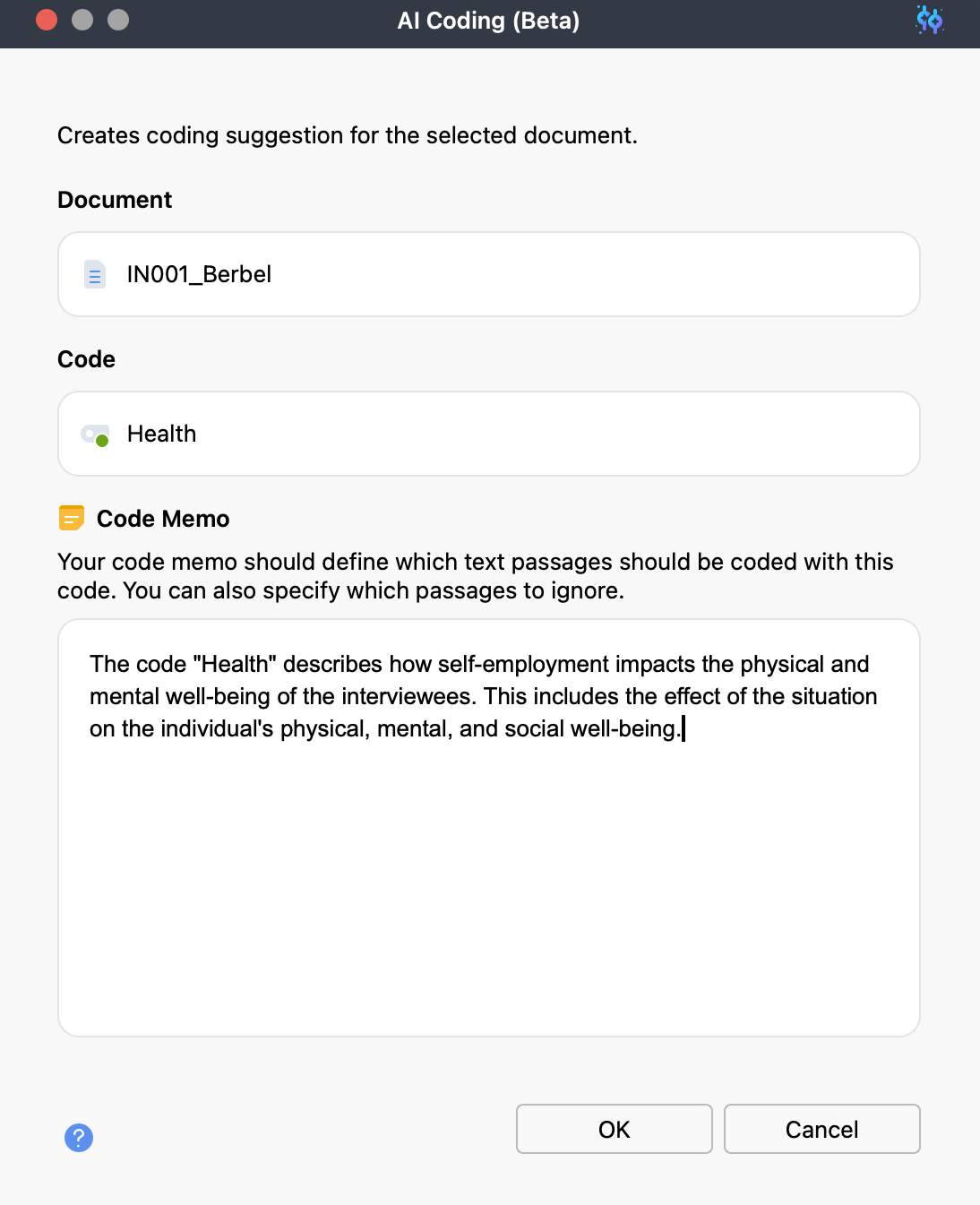
Example: Interface AI Coding
Once we click “OK”, the coding process will start. Shortly after, we will see our results:
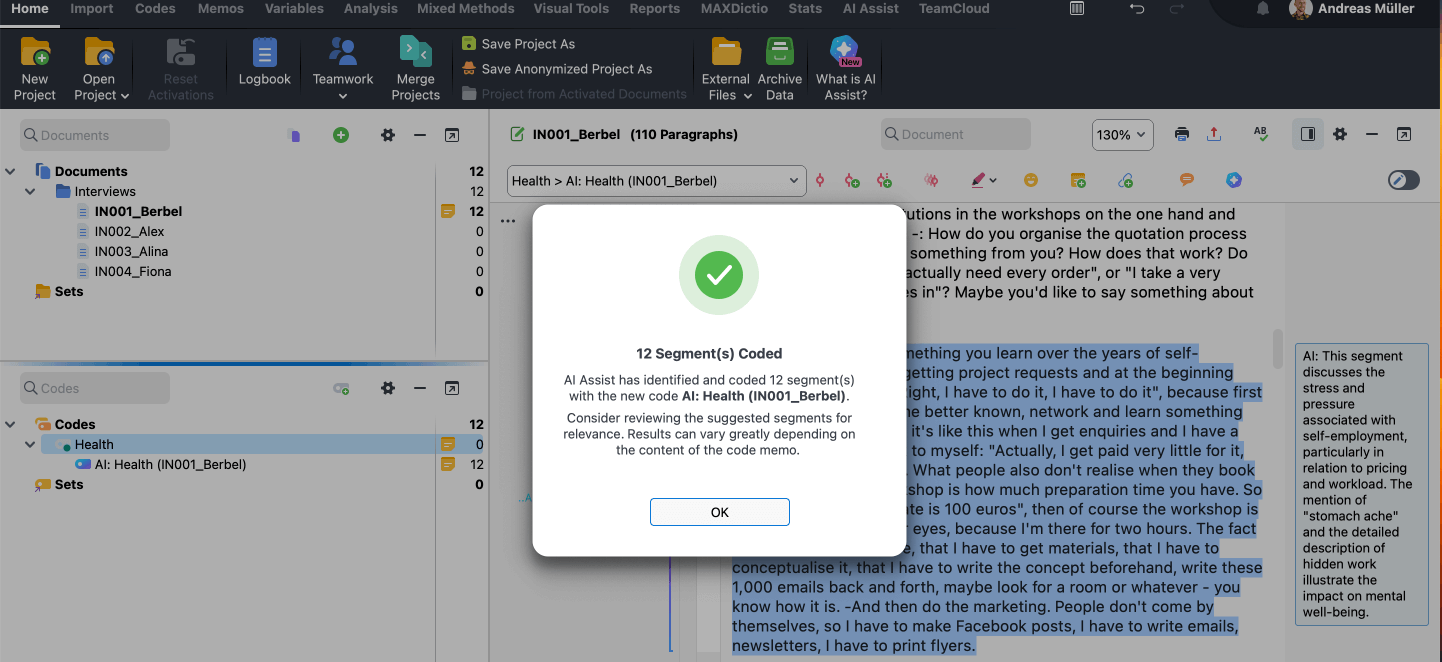
Coding Successful: 12 Segments were AI Coded.
As described above, the code is created as a subcode of our original code and marked with “AI:” and a special turquoise color. This way we can check our codes before we continue.
What makes a good code memo for AI Coding?
But what if we get no results? Or way too many?
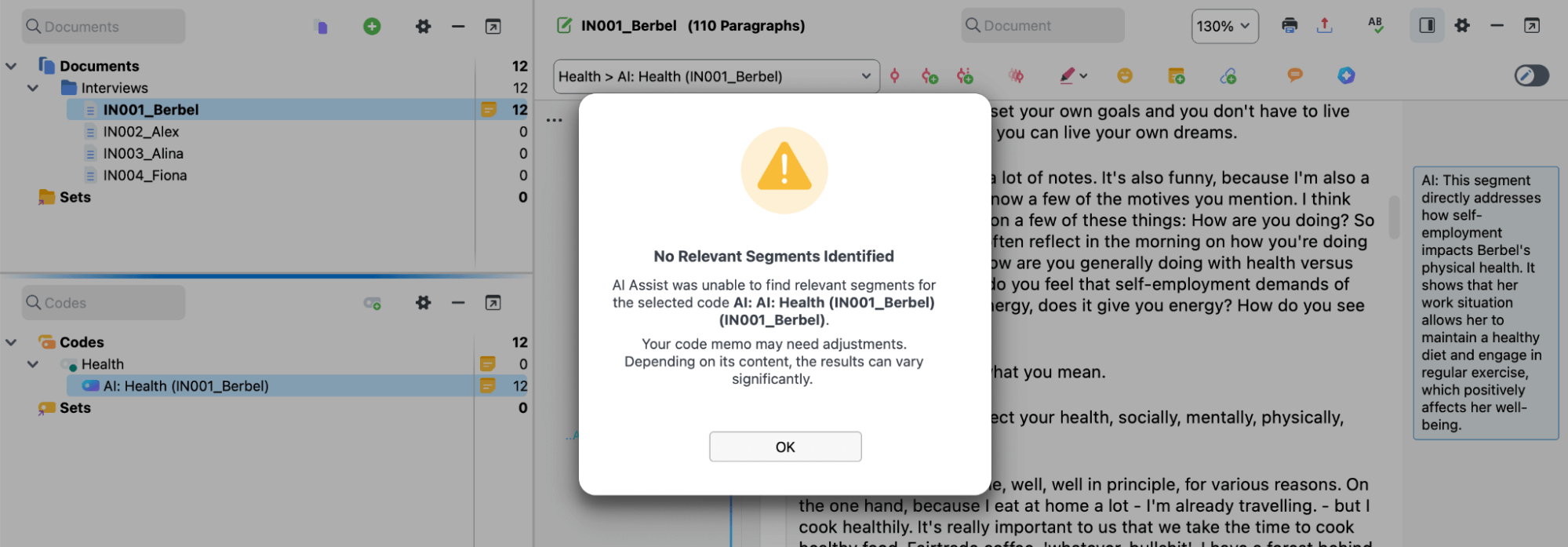
Error message: No retrieved segments identified.
Then it is high time to review our code memo. For AI coding to work, our code description must be explicit, precise, and a good fit for our data. We can also use exclusion and inclusion criteria to deliberately exclude certain content. Unlike manual coding, the use of examples should be avoided, as this can cause the AI to strongly prefer text segments that match the example.
How good are our initial AI coded results?
When we apply a newly created code with AI Coding for the first time, it is important to apply it to a document that we are familiar with. Knowing the data well is always helpful in evaluating the AI results, but especially in this early “testing” phase of the AI code and its description. At this stage, it pays to examine our coded data from two perspectives:
First, we verify that the coded segments actually contain the relevant data and do not contain unrelated data. We can do this by examining the coded segments. If we find unrelated or unwanted data, we can adjust our exclusion criteria for the code memo and rerun the AI coding.
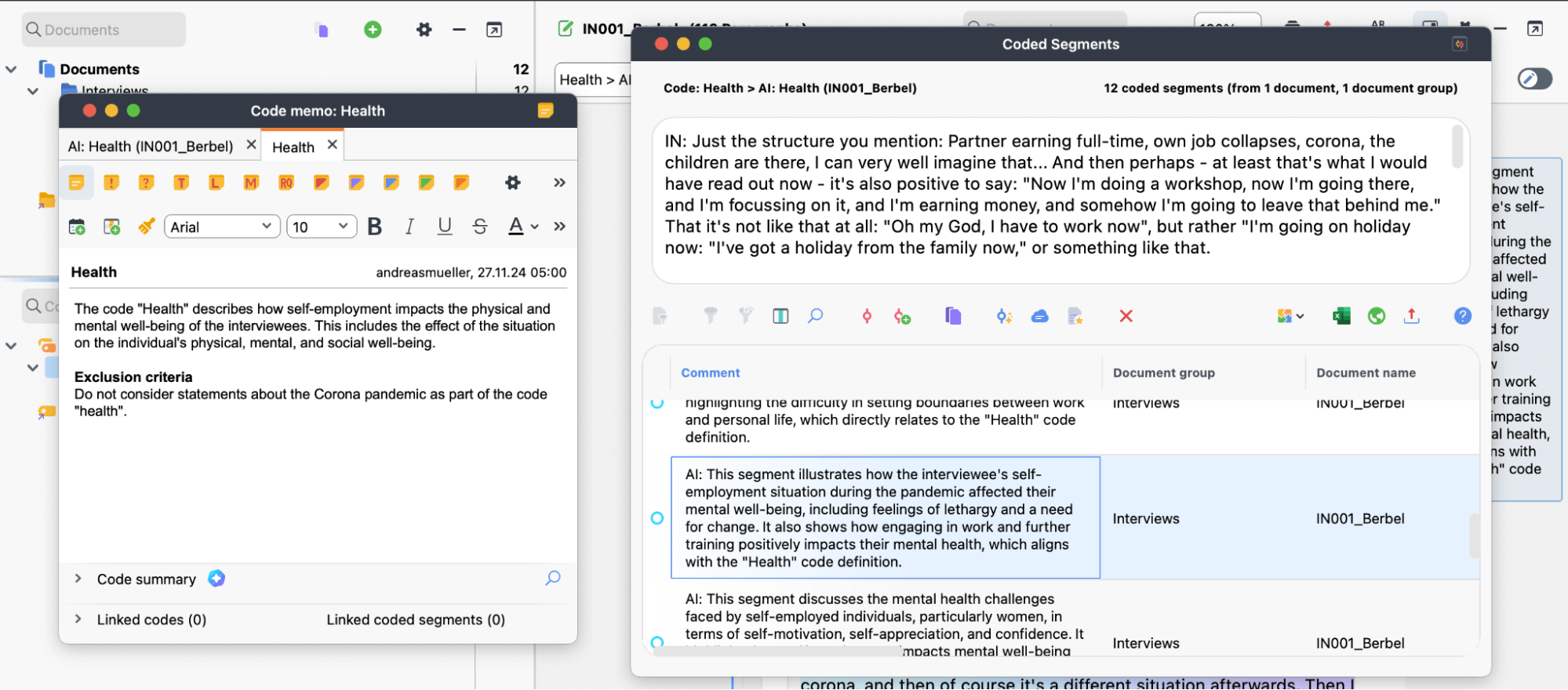
Overview of Coded Segments: Checking for segments that do not fit and adding exclusion criteria.
Second, we can go through the document and see if any important text segments have been left out. If so, we can adjust our inclusion criteria or the description of the code. It may help to add some words or phrases that also appear in the omitted segment.
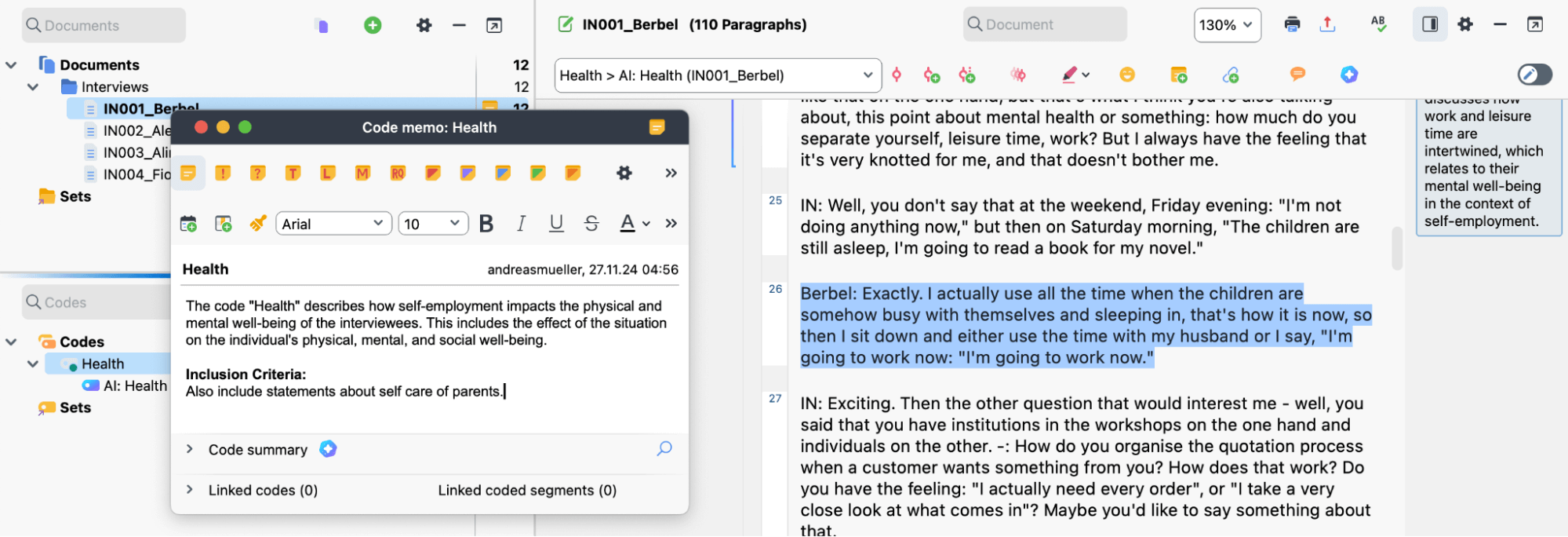
Example: Identifying a missing segment and adding an inclusion criteria.
Before we apply our code to multiple documents, we need to make sure that we test the code and the code memo well on one or a few documents that we are very familiar with. This will help us refine our code descriptions and ensure better results.
Step 2: Apply the codes to all the data
Once we are satisfied with the results of AI Coding on our initial documents, it is time to extend it to the entire dataset. To do this, we repeat the process described above: We select a document, run AI Coding, select the code from the code system, and apply the code to the data. Keep an eye on the code frequencies and review the results to make sure your code definition in the code memo fits the other documents well. If a document returns no coded segments (or too many of them), this is a good reason to investigate further. We can still adjust the code memo and even run it again on our initial set of documents to see how this change affects your earlier results. Once we have coded all of our documents with this code, our code system will look something like this:
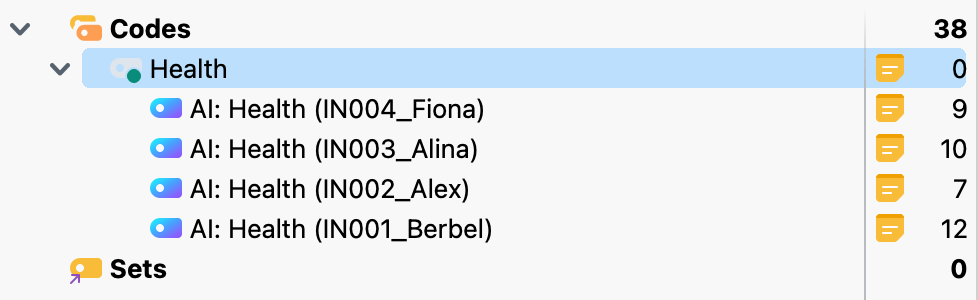
Example: Code System with four AI Codes regarding “Health”.
Each document has its own AI code as a subcode of the original parent code. Now we can merge all the AI codes into one. Just select all the codes, right click and choose “Merge Codes”.
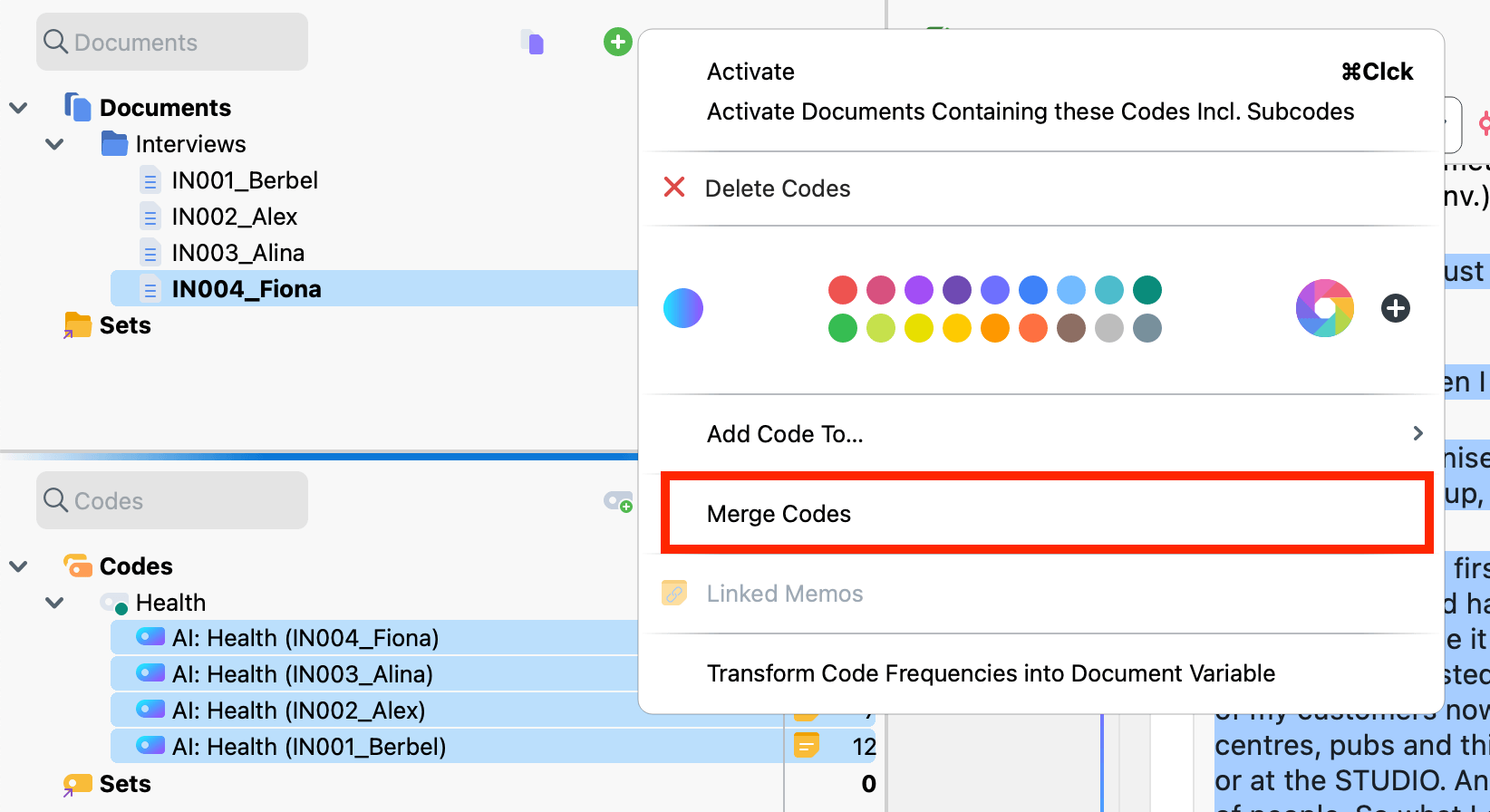
Now we can check our AI coded segments again. To do this, simply retrieve the data via the list of coded segments and verify that there are no incorrect coded segments:
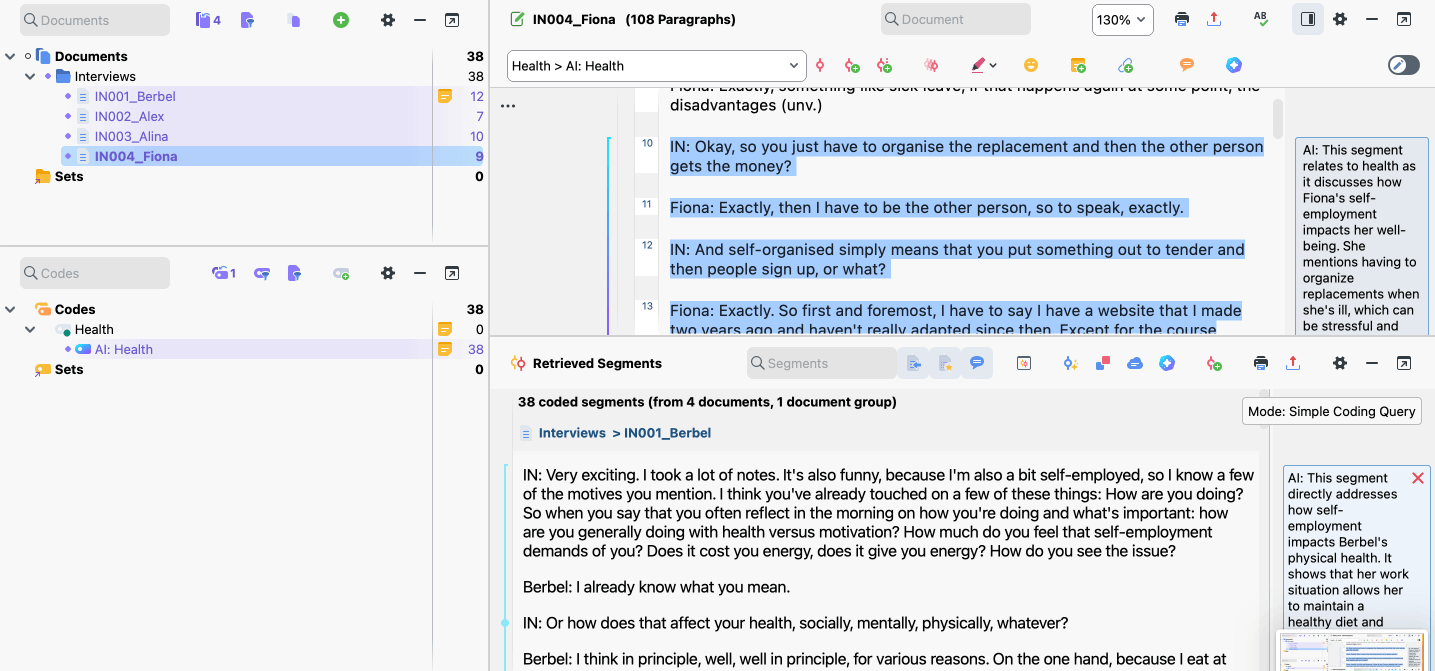
Reviewing coded segments via the list of retrieved segments
Once we have reviewed our coded segments, we can merge them into the main code along with any manual codes we may have created.
How do I use AI coding for more than one code?
Once we have coded all of our data with one code, it may be time to move on and create more codes. To do this, simply repeat the process described above. Create your code and code memo, test it on some data, and apply it to the rest of the data once you are done revising the code memo.
Using such a process, we don’t follow a “one-click” solution that magically produces the answer to your research questions. Instead, it is a very human-driven process. It requires us to be explicit about our codes and code definitions and to validate our results. It requires methodological rigor and training in how to create a codebook and how to judge the quality of coding. Far from making the researcher a simple user of a tool, the use of AI puts us in the role of a methods instructor and supervisor.
What challenges might I face with AI coding?
As streamlined as the process above may seem, we may of course run into problems along the way. When we use AI coding, two types of problems stand out:
First, we may not find any results. In this case, we want to adjust the code memo. Also, think about what this tells you about your data. For example, in a study focused primarily on the problems within an institution, a code “strength” may not yield many results because the current strengths of the system are rarely addressed in such problem-focused interviews. In this case, we can use text search (Analysis > Text Search and Auto-Code) to identify segments that are relevant to our code. We can then use these segments to refine our code memos.

The second challenge we may encounter is a technical limit on the amount of data the AI can handle with a single prompt or action. MAXQDA’s AI Assist can easily handle the transcripts of an hour-long interview. However, if the documents get much larger than that, we may receive an error message.
If our document is too long for AI Coding to code, we can try to shorten it. For example, interviews often have an introduction or conclusion that contains a lot of small talk that is not strictly necessary for the analysis. Trimming the beginning and end of a document in this way can help to get under the 120,000 character limit. Or, if you are working with document analysis, try to include only the part of the document that is actually relevant for the analysis. For example, you can remove the bibliography from a long publication before using AI Coding.
Step 3: Validating your AI coding using MAXQDA’s Visual tools
Once we have applied all of our codes to the entire dataset, we may want to evaluate the quality of our coding. Ideally, this would be done by manually checking each document as described for the subset of data in step 1. In larger projects, however, this may not be feasible and we will need a different strategy.
One of the great advantages of using AI as part of an established research software like MAXQDA is that we can use the wide range of features of the software in combination with AI: For example, we can use MAXQDA’s visual tools to evaluate the results of our AI coding for the entire dataset.
Code Matrix Browser: Have all my documents been coded?
For example, we can use “Visual Tools > Code Matrix Browser” to analyze the distribution of codes across different documents. In combination with AI coding, this can give us insight into whether our AI codes produced similar results across all documents, or whether some documents were left out.
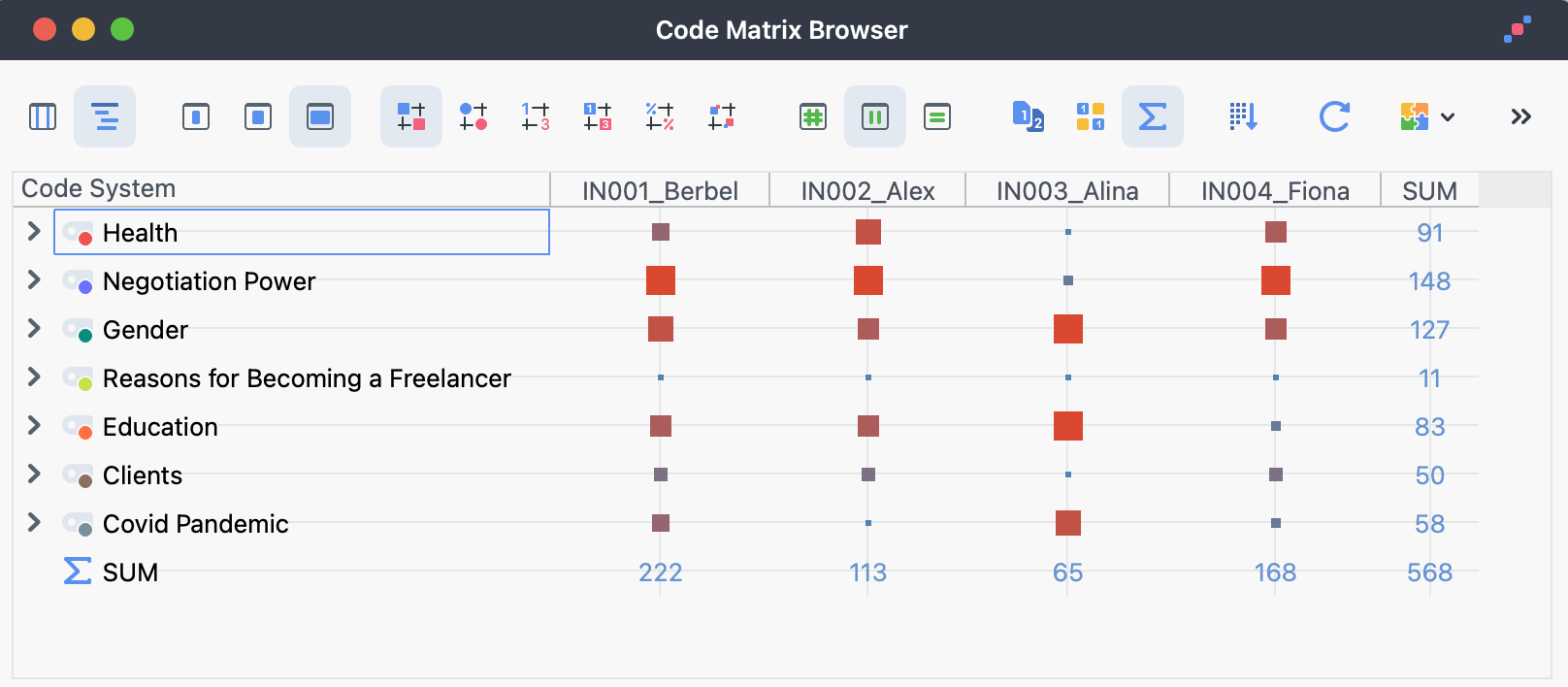
Code Matrix Browser: Distribution of AI Codes across the four documents
The example above shows the Code Matrix Browser. Larger squares indicate that the code was applied many times in a document, a smaller square indicates that the code was applied only a few times in that document. This can help us evaluate the quality of our coding. If a code produced very few or no results for a document, it may be worthwhile to re-examine the document and the code description.
When using such a visual tool, we should be especially careful not to take the code frequency, as reflected in the size of the squares, at face value. Since the size of the segments created by AI coding can vary widely, from a single sentence to several paragraphs, the numbers are not easily comparable (see Step 4 for more details).
Document Comparison Chart: What sections of the documents did the AI code?
In addition, we can use visual tools to check which sections within our documents were actually coded. To do this, we can use the Document Comparison Chart from the Visual Tools tab
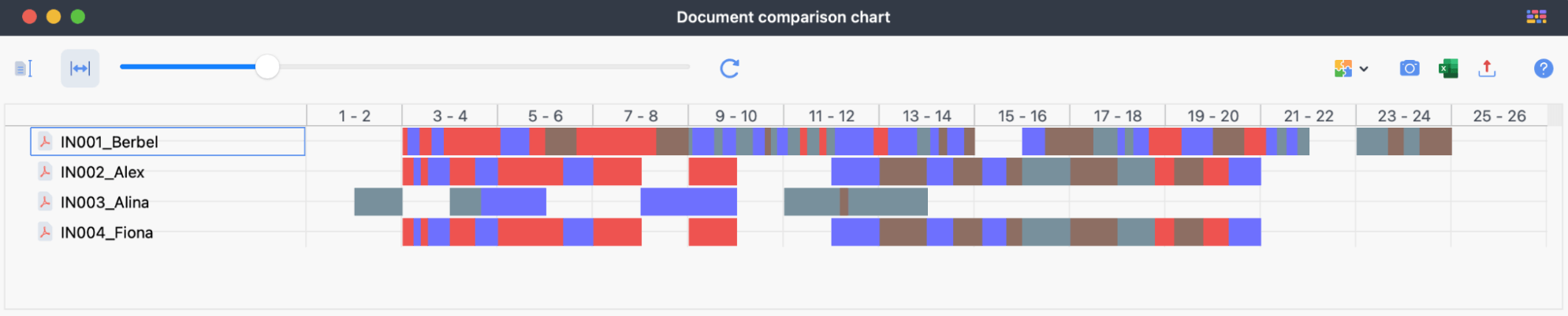
Document Comparison Chart: Showing that the beginning, paragraph 1-2, of most interviews has not been coded.
In the example above, we see a visualization of all our documents. Each row represents a document. The columns represent the different paragraphs within a document. The colors tell us what codes have been used in that section of the document. In this case, we can see that the beginning of the interviews was often not coded by the AI because it did not contain data directly relevant to the research question. In this way, we can quickly identify sections of the data that were not coded and investigate whether omitting that section serves the purpose of our research.
Step 4: Analyzing the AI Coded Data
Once we have reviewed all of our coded data, we can proceed with analysis. Here it may be interesting to analyze code frequencies, create code summaries, and use the Smart Coding tool.
Can I analyze the code frequencies of AI Coded data?
While it may be tempting to do so, we must be especially careful when quantifying our data, i.e., counting code frequencies or measuring code coverage (the area covered by a code). Although humans are not always good at being consistent, the size of code segments created by AI can vary quite a bit. The AI tries to identify units of meaning that are reflected in the coded segments. However, such a segment can range from a few words to several paragraphs. We can easily see this when we retrieve segments from the overview of coded segments and look at the “area” of a segment:
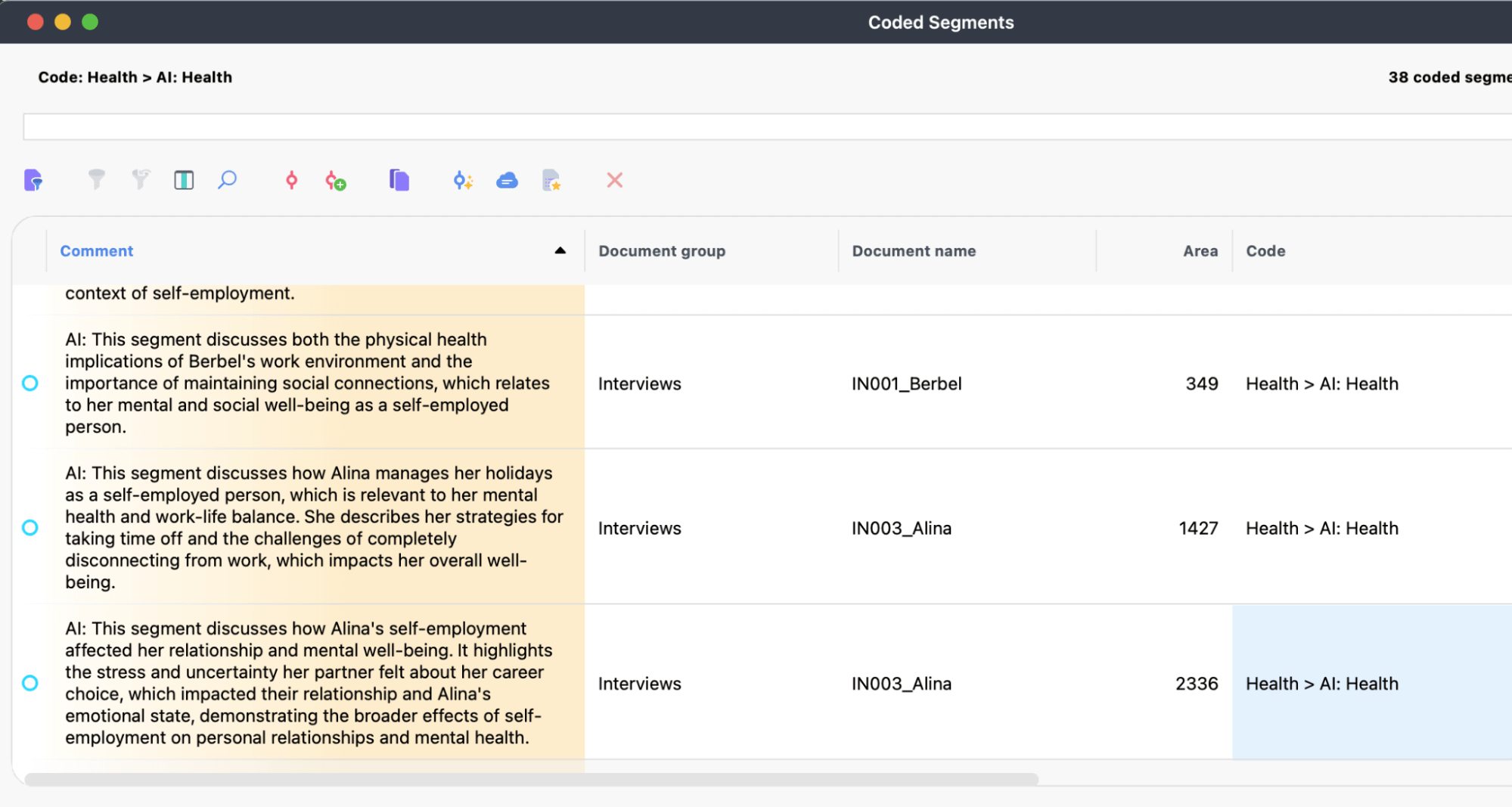
Overview of coded segments: Segments of different sizes, 349 characters, 1427 characters and 2336 characters long.
Similarly, when we rely heavily on AI encoding, we often read and consider only the encoded data selected by the AI. Although we are using our human reasoning, the AI may play an important role in deciding what text to read or not. In a study without AI, we usually read the entire text. Although we may end up coding only a small portion of it, the uncoded data is still included in the results, whether we are aware of it or not.
How do I summarize my coded data?
Once we have coded our data, we can also automatically create a summary for our coded data. This helps us summarize topics across documents. We can simply right click on a code and select “AI Assist > Summarize Coded Segments”. Next, we select the language and the desired length of the summary, and we get the following output:
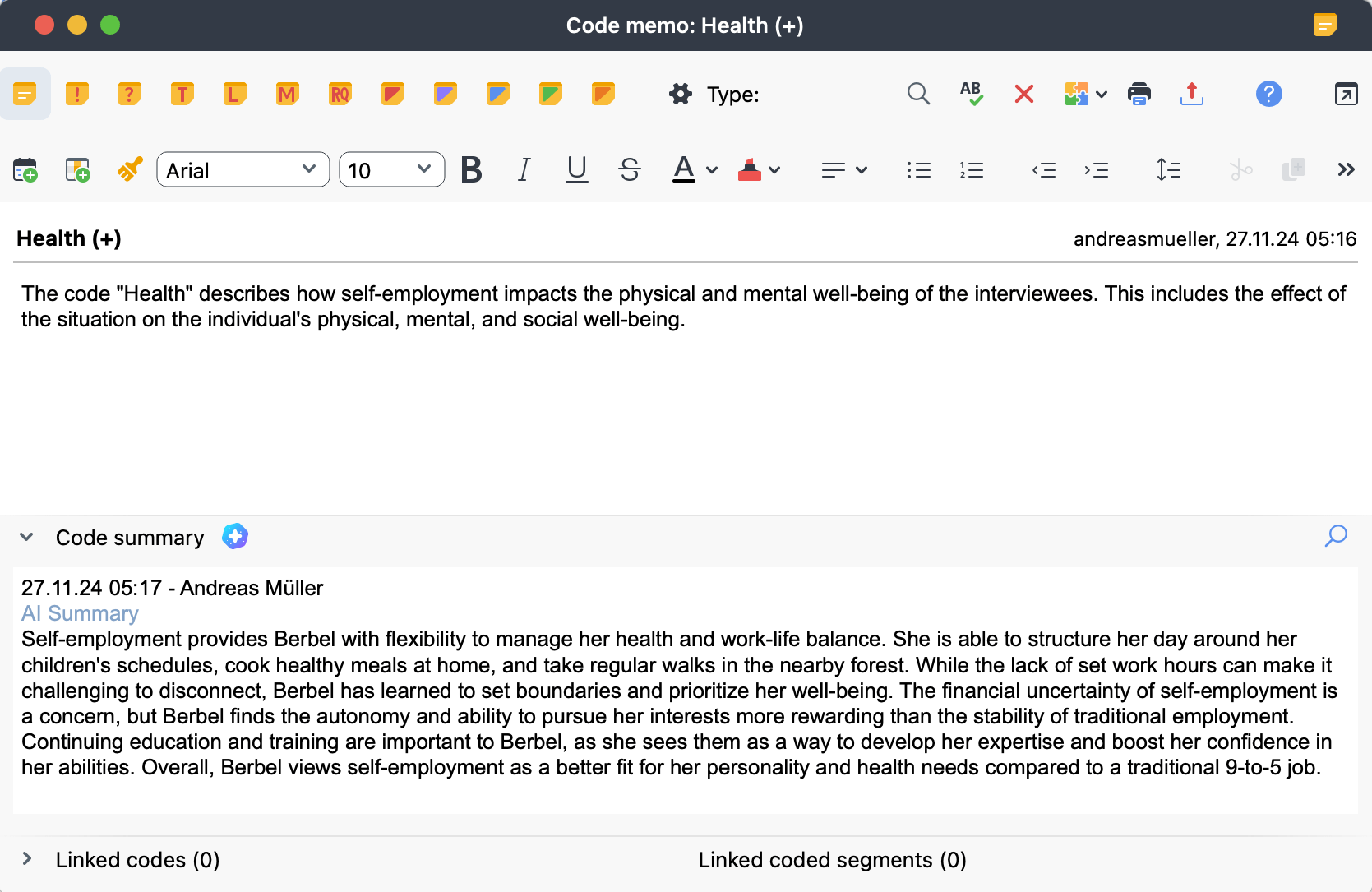
If the summary does not serve our purpose, we can also customize the summary with additional instructions:
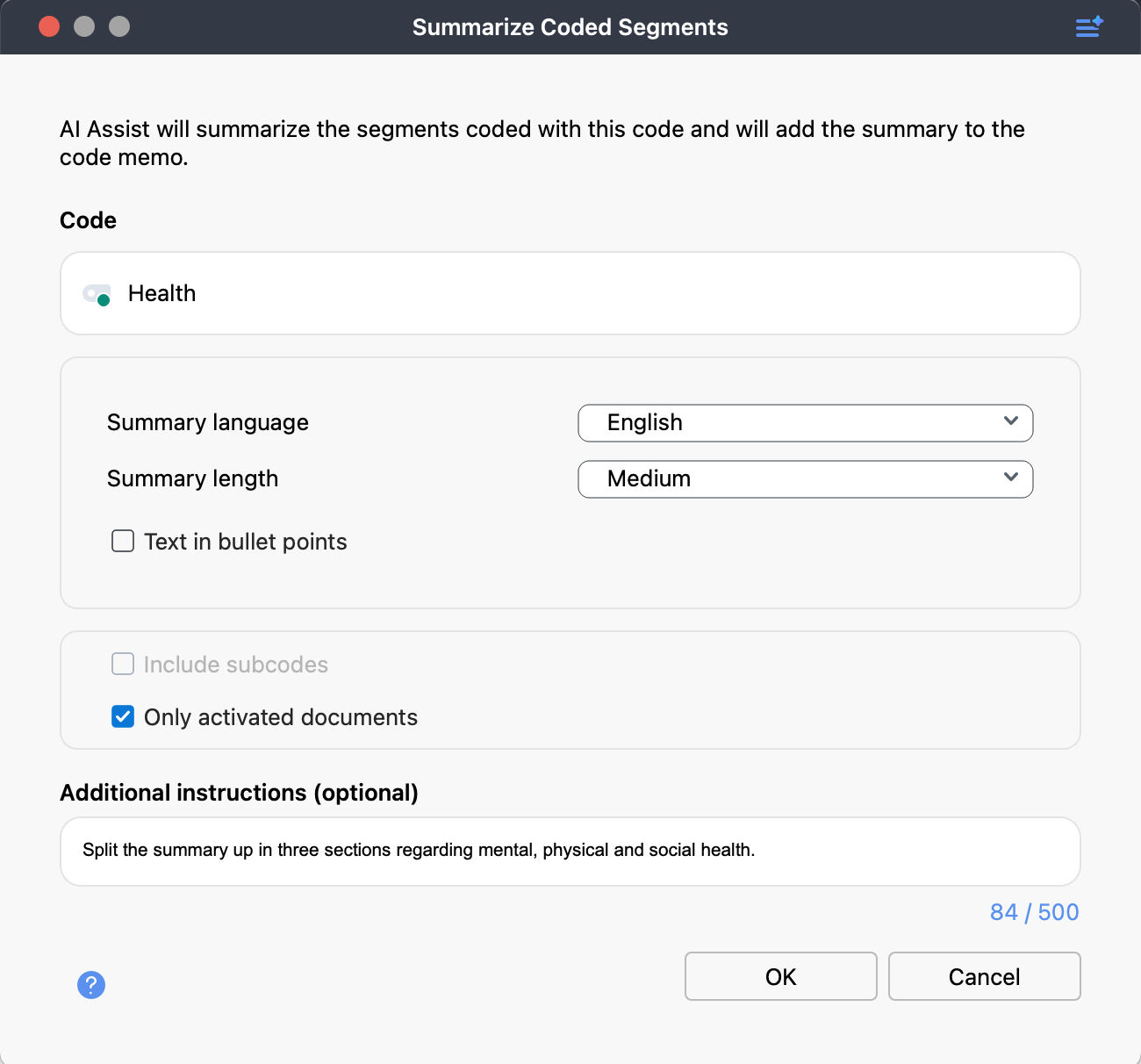
In the example above, we distinguish different facets within the coded data. For our code health, we request the AI to split the summary up into three sections regarding mental, physical and social health.
The Smart Coding Tool: How to Create Subcodes?
Since AI coding often creates codes with a large number of coded segments. It is an interesting option to continue working with the Smart Coding tool:
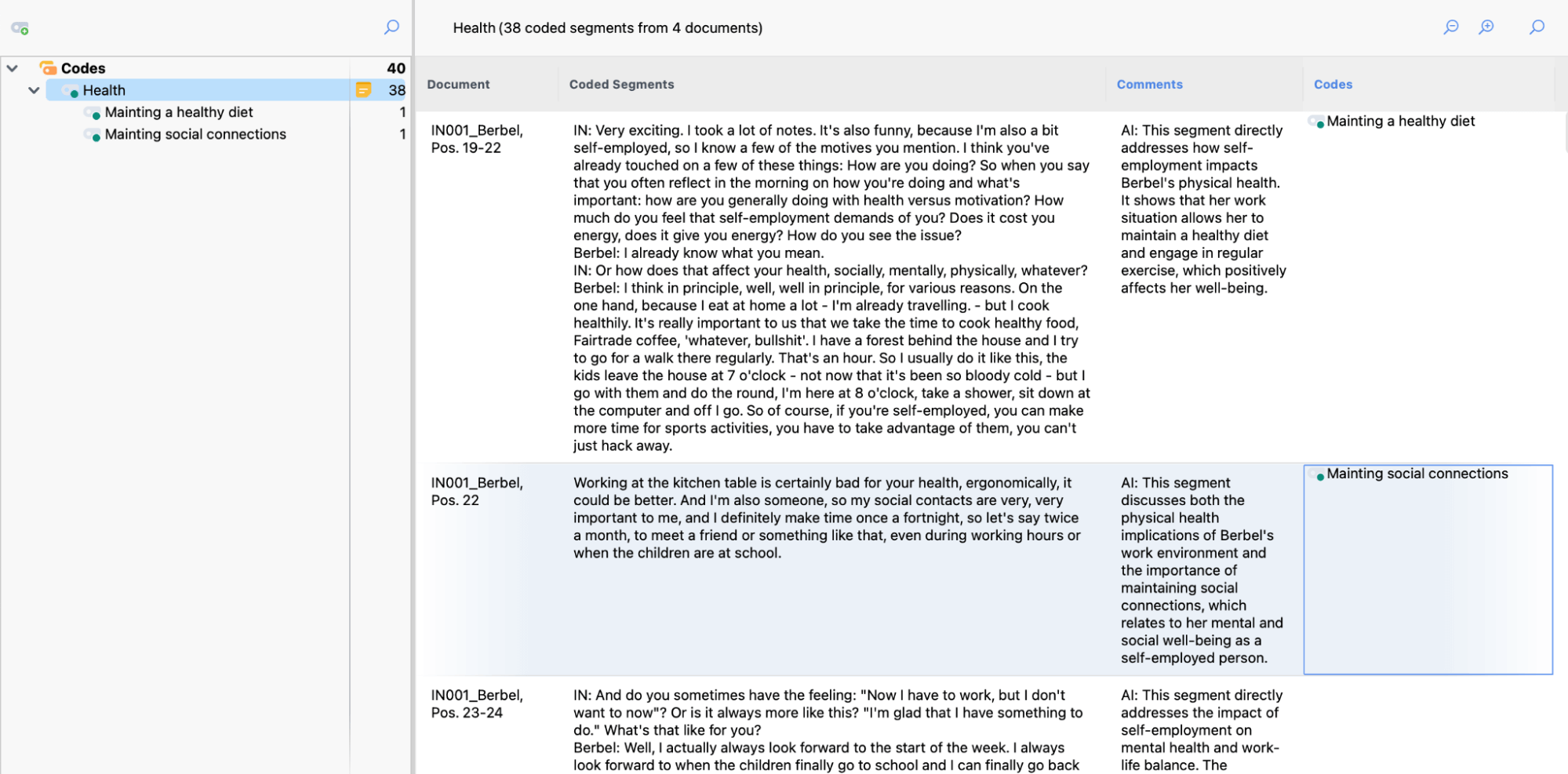
Example: Splitting up the code “health” into different actions such as “mainting a healthy diet” or “mainting social connections”.
The Smart Coding tool (Codes > Smart Coding) allows us to comprehensively review all the contents of a code. As we can see in the screenshot above, it brings together all coded segments, their comments and other applied codes in one place. As a next step, we can go through our data code by code, segment by segment, creating and applying new subcodes for each code.
To do this, we simply read a segment and try to come up with an appropriate subcode. We then click on the green “+” icon next to the main code. We enter a code name and then drag and drop the selected coded segment onto the (new) code. To come up with good subcode names, we can review the segment comments created earlier by the AI, or we can ask the AI for subcode suggestions (AI Assist > Suggest Subcodes).
This step is crucial, as it not only helps to create a useful subcode system that fits our research question, but also ensures that every single segment coded by an AI is reviewed at least once by a human researcher. By following such a process of initially applying deductive, concept-driven codes and then refining them with inductive, text-driven subcodes, we also follow the method of qualitative content analysis as proposed by Kuckartz & Rädiker (2023).
Conclusion: AI and human coding a complex relationship
In conclusion, we will take a look at three key questions regarding the differences between human and AI coding, the use cases for AI coding, and the potential impact of AI coding on qualitative research.
How is AI coding different from human coding?
As we have seen, the process applied here fits well with established methods. Of course, we have to be extra careful about quantifying our codes and missing relevant segments, but these are also shortcomings in many non-AI projects.
For this type of approach, we see a major methodological difference: AI coding is deductive or concept-driven to the extreme. As human researchers, we never just focus on a single code. When we read a text and try to identify different concepts, we always weigh each segment against the options of several existing codes or even potential future codes. We may find a text segment that does not fit into our system, but we may still consider it and allow it to influence our code system. AI coding, as applied here, works the other way around: It takes a description and looks only for what fits that specific description.
However, we must keep in mind that this does not apply to all possible applications of AI Coding. For example, if we use “Suggest codes for selected segment” another tool from the “AI Assist” tab, we are at the other end of the extreme: only new codes are suggested based on the text, without taking into account our previous codings or findings.
What is a good use case for qualitative AI coding?
When we think about practical use cases for AI coding, we see it as both enhancing human coding and extending it to larger amounts of data. First, AI coding can serve as a fast and inexpensive second coder. As qualitative researchers, we are trained to constantly evaluate the subjectivity of our analytical work, and we might as well be haunted forever by the grain of doubt that we might have missed that one important segment. AI coding allows us to challenge our human subjectivity and complement it with the subjectivity of an AI model. It can help us point out segments of text that we may have overlooked or discarded too quickly. Here, AI can be used as a mirror to critically evaluate our own coding.
Second, we can also use AI coding to overcome the challenge of handling larger amounts of data. If we have already manually coded a substantial example dataset, we can use this knowledge and existing code descriptions as an ideal basis for extending our codes to a larger dataset. MAXQDA’s visual tools are a great help here, as they allow us to verify our coding results even for larger data sets.
What can AI coding bring to qualitative research?
Of course, AI coding will be used – and probably already is being used – to produce “quick and dirty” results to get a thesis passed or a paper submitted, and the danger of polluting the seemingly endless ocean of research results with the few substantive “findings” from “AI studies” is a serious one. However, our most hopeful vision of the use of AI in qualitative research, however, is this: It can help us challenge our subjectivity and human biases even further. Due to resource constraints, few projects can ever afford extensive inter-coder agreement checks, or even just a second opinion on the same data. With the use of AI, we have quick access to a second “opinion” that can help us improve not only the quantity of results, but also the quality of our research findings.
Author:
![]()
Andreas Müller has been a professional MAXQDA trainer for over seven years, and is a research and methods coach and contractual researcher. He is experienced in a wide array of qualitative methods and works with clients from diverse academic disciplines, including healthcare, educational sciences, and economics. Andreas specializes in the analysis of qualitative text and video data, as well as mixed methods data.
www.muellermixedmethods.com
Literature
Braun, Virginia; Clarke, Victoria: Thematic Analysis: A Practical Guide. Strauss, Anselm; Corbin, Juliet (1990). Basics of qualitative research: Grounded theory procedures and techniques. Thousand Oaks, CA: Sage.
Kuckartz, Udo; Stefan Rädiker (2023): Qualitative Content Analysis: Methods, Practice and Software. SAGE Publications Ltd.
Müller, Andreas;. Rädiker, Stefan (2024). “Chatting” With Your Data: 10 Prompts for Analyzing Interviews With MAXQDA’s AI Assist. MAXQDA Research Blog. https://www.maxqda.com/blogpost/chatting-with-your-data-10-prompts-for-analyzing-interviews-with-maxqda-ai-assist
Rädiker, Stefan et.al (2024): AI in Research: Opportunities and Challenges. (2024, February 29). [Symposium]. MAXQDA International Conference (MQIC), Berlin, Germany. https://www.maxqda.com/blogpost/ai-in-research-opportunities-and-challenges